A Revolution in Patient Data Analysis
4 minute read
Today we are going to look into Multi-Modal
Learning, and how it can help healthcare professionals who are faced with an
overwhelming amount of patient data. Electronic health records, medical
imaging, genomic data, and wearable devices generate a massive stream of
information. The challenge lies in harnessing this data to improve patient
care, diagnosis, and treatment. Multi-modal learning, a cutting-edge approach
in artificial intelligence (AI), is emerging as a game-changer in healthcare.
This blog post explores the power of multi-modal learning and its applications
in analyzing patient data.
What is Multi-Modal Learning?
Multi-modal learning is a machine learning
technique that combines information from various data sources or modalities to
improve the performance of a model. In healthcare, these modalities can include
text data from electronic health records, medical images, genomic sequences,
sensor data from wearables, and more. By integrating and analyzing data from
multiple sources, multi-modal learning offers a comprehensive view of a
patient's health and can lead to more accurate and personalized healthcare
solutions.
Examples of Multi-Modal Learning in Healthcare
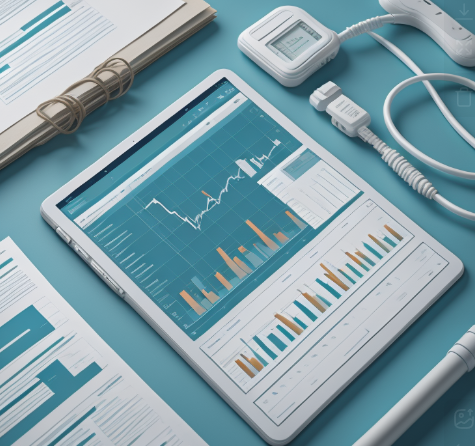
1. Disease Diagnosis and Prediction
One of the most promising applications of
multi-modal learning in healthcare is disease diagnosis and prediction. Imagine
a patient who visits a healthcare facility with symptoms that are difficult to
diagnose based solely on medical imaging or clinical history. Multi-modal
learning can help by combining various sources of data.
For instance, a patient's medical history,
lab test results, and radiology images can be integrated using a multi-modal
learning model. This approach can provide a more accurate diagnosis, leading to
timely and targeted treatment.
2. Medical Image Analysis
Medical imaging plays a crucial role in
diagnosing and monitoring various health conditions. Multi-modal learning can
enhance the accuracy of medical image analysis by combining different types of
imaging data. For example, combining MRI scans, CT scans, and ultrasound images
can improve the diagnosis of complex conditions like brain tumors or liver
diseases.
Furthermore, multi-modal learning can help
in the early detection of diseases. For instance, a multi-modal model can
analyze mammograms, patient history, and genetic information to identify
individuals at high risk for breast cancer, leading to early intervention and
better outcomes.
3. Personalized Treatment Plans
Personalized medicine is an evolving
approach in healthcare, and multi-modal learning can be a key enabler. By
integrating data from various sources, including genetic information, patient
history, and real-time sensor data from wearable devices, healthcare providers
can tailor treatment plans to individual patients.
For example, for a patient with diabetes, a
multi-modal model can consider genetic predisposition, lifestyle data from
fitness trackers, and real-time blood glucose levels to develop a personalized
management plan. This approach can significantly improve the patient's quality
of life and treatment outcomes.
4. Predictive Healthcare Analytics
Healthcare institutions and providers can
benefit from multi-modal learning for predictive analytics. By analyzing a
diverse set of data, including patient records, clinical notes, and sensor
data, healthcare organizations can predict disease outbreaks, patient
admissions, and resource allocation needs more accurately. This can lead to
more efficient healthcare delivery and better patient care.
Challenges in Multi-Modal Learning
While multi-modal learning holds great
promise, it comes with several challenges, especially in the healthcare domain:
1. Data Integration: Integrating data from
various sources and modalities can be complex. Data pre-processing and feature
engineering are critical steps in ensuring data compatibility and consistency.
2. Privacy and Security: Healthcare data is
highly sensitive, and maintaining patient privacy is paramount. Secure data
sharing and privacy-preserving techniques are essential in multi-modal learning
applications.
3. Interpretable Models: Interpreting the
results of multi-modal models can be challenging. Healthcare professionals need
to understand and trust the model's decisions for it to be widely adopted.
4. Data Quality: The accuracy and quality
of data from different modalities can vary significantly. Noisy or erroneous
data can negatively impact the performance of multi-modal models.
Conclusion
Multi-modal learning is poised to
revolutionize the way healthcare professionals analyze patient data. By
combining information from various sources, it offers a more comprehensive and
accurate understanding of a patient's health. From disease diagnosis and
prediction to personalized treatment plans and predictive analytics, the
applications are diverse and promising.
However, addressing challenges such as data
integration, privacy, interpretability, and data quality is crucial for the
successful implementation of multi-modal learning in healthcare. As these
challenges are overcome, multi-modal learning will become an indispensable tool
in delivering more precise and personalized healthcare, ultimately improving
patient outcomes and the quality of healthcare services.
Speak to Qvantia today, we would be very happy to help - info@qvantia.com
Qvantia - AI Insights