A 6 min read
The landscape of healthcare is undergoing a seismic shift, fuelled
by the burgeoning field of Artificial Intelligence. AI is demonstrating remarkable potential in
revolutionizing disease diagnosis, prognosis, and treatment. One of the most
exciting frontiers in this revolution is the use of AI to predict disease advancements,
offering a powerful tool for early intervention and improved patient outcomes. Continue reading to learn how AI Predicts Disease Advancement.
The Power of Data: Unlocking Insights with AI
At the heart of AI's power lies data. The healthcare
industry is awash in data, from electronic health records (EHRs) and medical
imaging to genomic sequencing and wearable sensor data. This wealth of
information, however, is often siloed, making it challenging to extract
meaningful insights.
This is where AI shines. Sophisticated algorithms can
analyze vast and disparate datasets, identifying complex patterns and
correlations invisible to the human eye. Combining diverse primary data sets,
such as:
These allow AI to paint a comprehensive picture of an
individual's health status. By extracting common features and identifying
subtle changes over time, AI can predict disease advancement with remarkable
accuracy, often before traditional methods can detect any signs.
Early Detection, Better Outcomes: Two Powerful Use Cases
Ignoring drift is like
sailing blindfolded. Without awareness
of the changing landscape, your AI models are destined to falter, leading to:
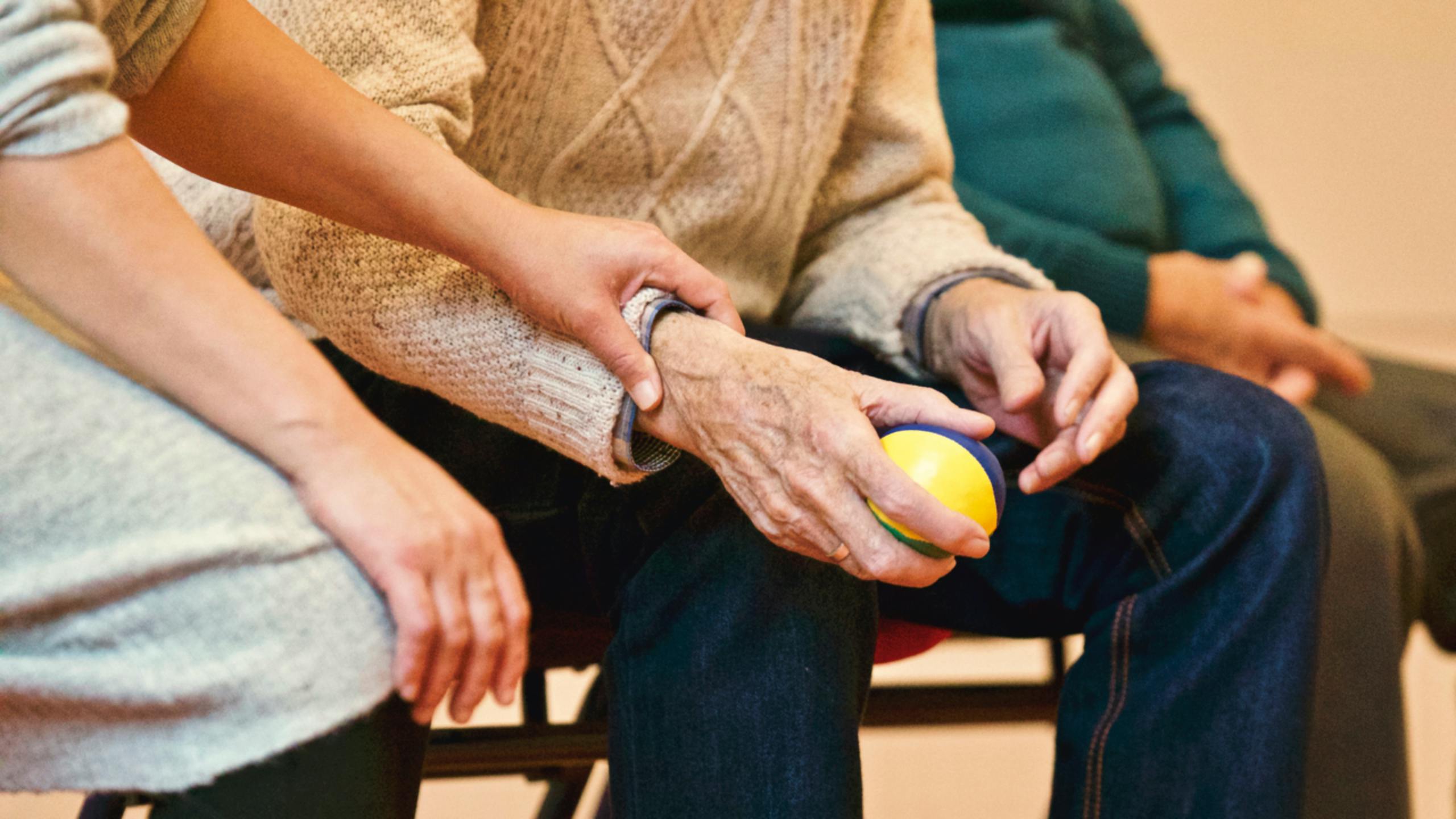
1 - Alzheimer's Disease: This neurodegenerative
disease is notoriously difficult to diagnose early, often manifesting
clinically only after significant cognitive decline. AI algorithms, however,
are proving invaluable in identifying early warning signs.
Process: Researchers
are training AI models on large datasets of brain scans (MRI, PET), genetic
information, and cognitive test results from both healthy individuals and those
with Alzheimer's.
Early Detection: The
AI can then analyze new patient data, identifying subtle changes in brain
structure, function, and biomarkers that indicate an increased risk of
developing Alzheimer's, potentially years before symptoms emerge. This early
detection opens a crucial window for interventions that can delay or
potentially prevent the onset of debilitating symptoms.
2 - Cardiovascular Disease: A leading cause of death
worldwide, cardiovascular disease often progresses silently until a
catastrophic event like a heart attack or stroke. AI offers a proactive
approach to risk assessment and early intervention.
Process: AI models are trained on vast datasets incorporating patient demographics, medical history, lifestyle factors, and biomarkers like cholesterol levels and blood pressure readings.
Early Detection: Analyzing this data, AI algorithms can identify individuals at high risk of developing cardiovascular disease, even if their traditional risk factors seem moderate. This early warning enables personalised interventions, such as lifestyle modifications, medication, and closer monitoring, to mitigate the risk and improve long-term outcomes.
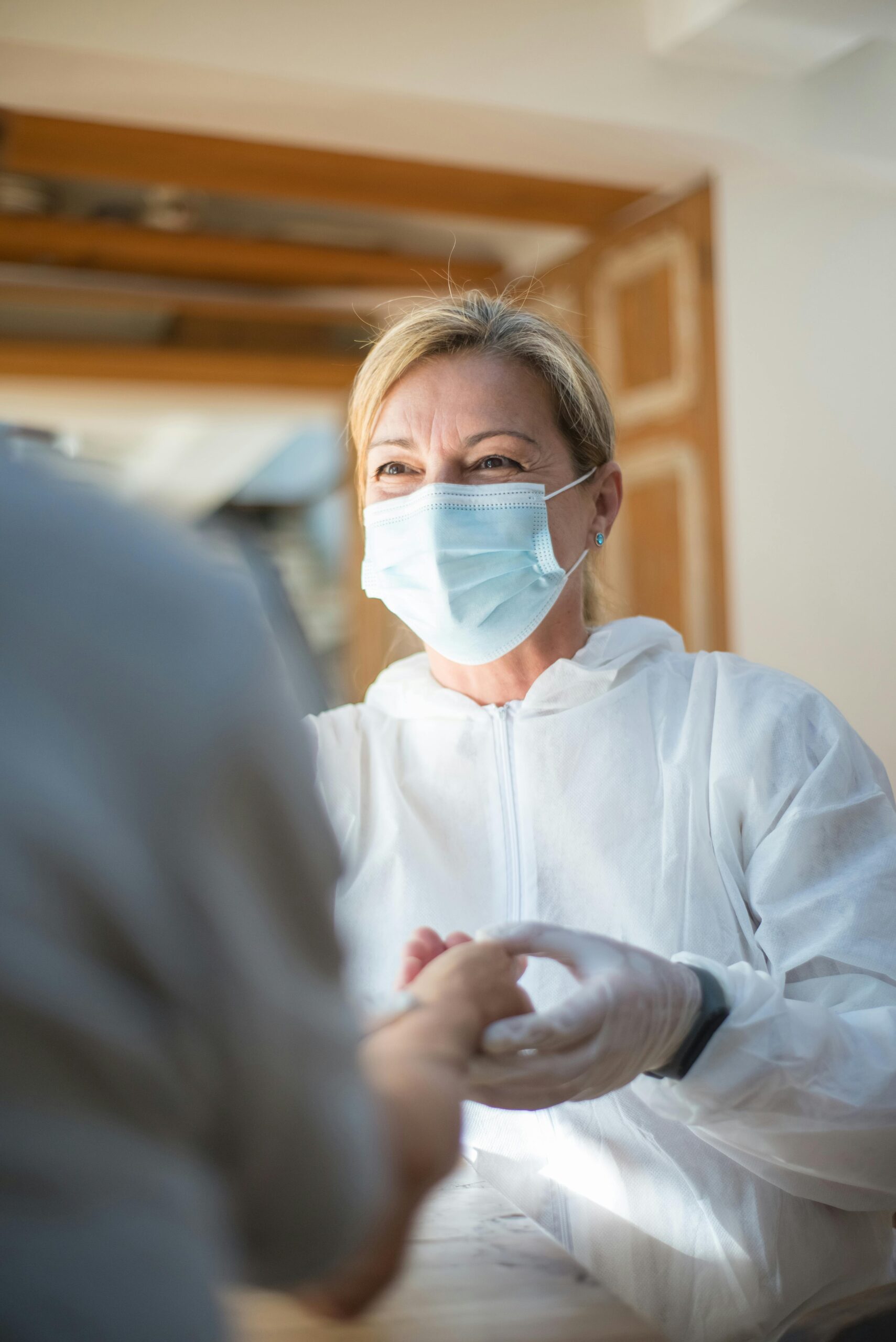
The Path Forward: From Prediction to Prevention
The potential of AI in predicting disease progression is
vast and rapidly expanding. The process generally involves:
Data Acquisition and Preparation: Gathering diverse and relevant datasets, cleaning and preprocessing the data to ensure accuracy and consistency.
Model Training: Using machine learning algorithms to train AI models on labeled datasets, teaching the AI to recognize patterns associated with disease progression.
Model Validation: Testing the trained AI model on independent datasets to evaluate its accuracy and generalizability.
Clinical Implementation: Integrating
the validated AI tool into clinical workflows, providing physicians with
actionable insights to guide patient care.
And now, thanks to Qvantia’s no-code solution, the speed at
which these advancements are being made has shifted up several gears. Not only can every day users
Qvantia - AI Insights