A 6 min read
The world of AI is
exhilarating. We're witnessing machines
mimic human intelligence, automating tasks and making decisions with remarkable
accuracy. Yet, behind this alluring
facade lies a silent threat, eroding the very foundation of AI's
effectiveness: Drift.
Imagine this: you've meticulously trained a model to predict customer churn with impressive precision. You deploy it, feeling confident about its ability to provide actionable insights. However, weeks later, you notice the predictions becoming increasingly inaccurate. What went wrong?
The answer likely lies
in data drift. Your model,
trained on a specific snapshot of data, is now encountering a reality that has
shifted. This drift can manifest in
various forms, affecting both the incoming data and the model's performance
over time.
The Two Faces of
Drift: Data and Model Degradation
Data drift occurs when the statistical properties of
the input data change over time. This could be due to several factors:
Evolving Consumer Behaviour: Take the example of an e-commerce recommendation system. Seasonal
trends, new product launches, or even economic downturns can dramatically alter
customer preferences and purchasing patterns.
Changing External Factors: A model trained to predict traffic flow pre-pandemic will likely
falter when faced with the altered commuting patterns post-pandemic.
Data Quality Issues: Errors
in data collection, sensor malfunctions, or changes in data sources can
introduce inconsistencies that lead to drift.
Model drift, on the other hand, refers to the gradual decline in a model's predictive power over time. This can be a consequence of data drift or due to:
Concept Drift: This
occurs when the relationship between the input features and the target variable
evolves. For example, a model trained to identify spam emails based on certain
keywords might become less effective as spammers change their tactics.
Model Complexity: Overly
complex models, prone to overfitting on the training data, might struggle to
generalize well to new data patterns.
Why Drift Detection
is Not Optional
Ignoring drift is like
sailing blindfolded. Without awareness
of the changing landscape, your AI models are destined to falter, leading to:
Inaccurate Predictions and Decisions: A model suffering from drift delivers unreliable predictions,
potentially leading to poor business decisions and financial losses.
Eroded Trust in AI: Inaccurate
predictions erode trust in AI systems, making stakeholders hesitant to adopt
and invest in future AI initiatives.
Missed Opportunities: Anundetected drift might mask valuable insights and emerging patterns within the
data, hindering your ability to adapt to changing circumstances.
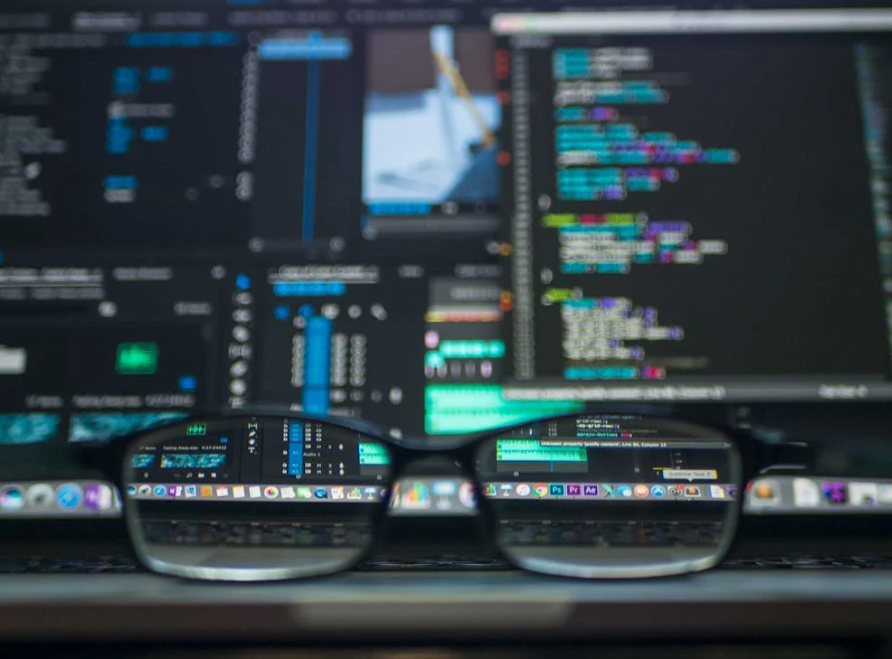
The Power of
Proactive Monitoring: Implementing Drift Detection
Drift detection is not a one-time activity but an ongoing process that requires constant vigilance. Here's how you can implement it:
Establish Baseline Performance: Before deployment, establish a clear understanding of your model's
expected performance using relevant metrics like accuracy, precision, and
recall.
Monitor Data Quality: Implement
data quality checks to identify anomalies, missing values, and inconsistencies
in the incoming data stream.
Statistical Monitoring: Utilize
statistical techniques like:
Population Stability Index (PSI): Measures the difference in distribution between two datasets
(e.g., training data and new data).
Kolmogorov-Smirnov Test (KS Test): Compares the cumulative distributions of two datasets to detect
significant differences.
Drift Detection Methods (DDM): Algorithms specifically designed to detect changes in data streams
by analyzing data distribution or model prediction errors.
Performance Monitoring: Continuously
track your model's performance metrics in the real-world environment. If you
observe a significant deviation from the established baseline, it's a strong
indication of drift.
Visualizations: Utilize
data visualization techniques to gain a visual understanding of data
distributions and model performance over time. This can help identify trends
and anomalies that might not be apparent from numerical metrics alone.
Qvantia has an array of different AI solutions which address the many challenges of Drift. Speak to us today to find out more.
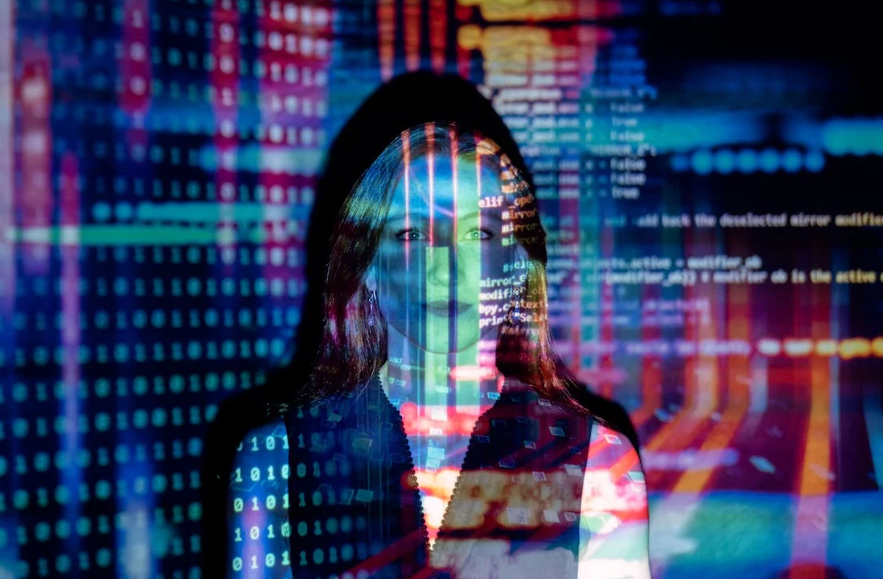
Addressing Drift:
Maintaining Model Relevance
Detecting drift is
only half the battle. Once identified, you need to take corrective actions:
Data Re-evaluation and Pre-processing: Analyze the drifted data to understand the cause. Address data
quality issues, update data pre-processing steps, or consider collecting new
data that reflects the current reality.
Model Retraining: Retrain
your model on a fresh dataset that includes the recent data reflecting the
observed drift. This helps the model adapt to the new patterns and
relationships within the data. This is why AI Drift in models
Model Update or Replacement: If retraining proves ineffective, consider updating the model architecture or even replacing it with a more suitable alternative based on the nature of the drift.
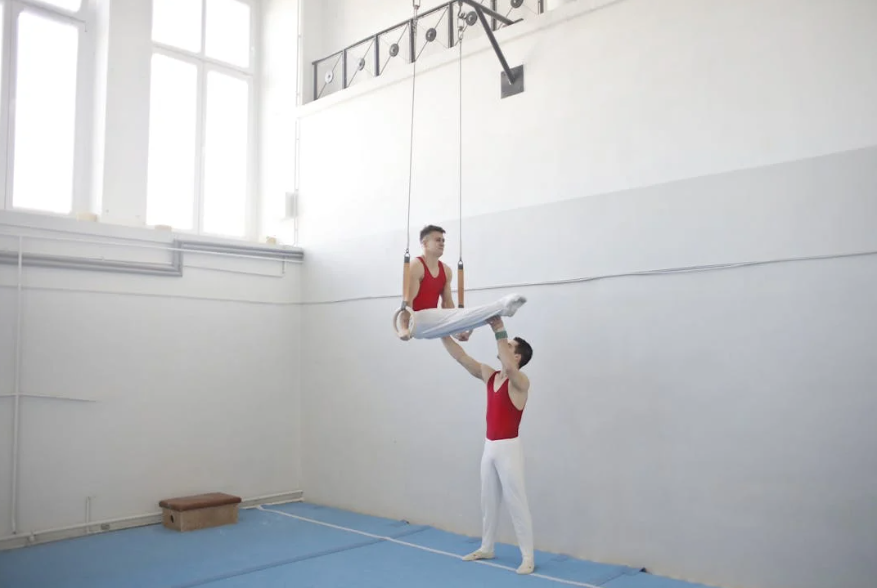
Conclusion:
Embracing Drift as a Constant Companion
In the ever-changing
world of data, drift is not an anomaly but an inherent characteristic. By
acknowledging its presence and implementing robust drift detection mechanisms,
you transform it from a threat to an opportunity. Proactive monitoring and
adaptation empower your AI models to remain relevant, reliable, and capable of
delivering impactful results in a dynamic environment.
Speak to Qvantia today, we would be very happy to help - info@qvantia.com
Qvantia - AI Insights